ASNC is your source for the latest information emerging from the field of nuclear cardiology and cardiac imaging.
From our flagship scientific journal to our weekly newsletter, ASNC is dedicated to delivering high-quality research, news, and updates from the field.
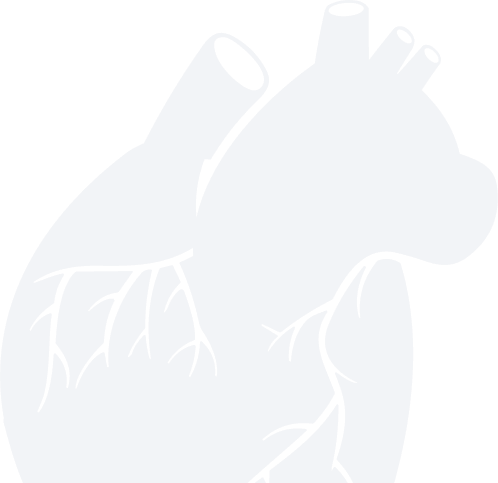
Journal of Nuclear Cardiology
The Journal of Nuclear Cardiology (JNC) is the only journal in the world devoted to this dynamic and growing subspecialty. The JNC is the official peer-reviewed publication of ASNC featuring the latest research findings and clinical developments in nuclear cardiology and related fields.
Read the latest issue of the Journal of Nuclear Cardiology (JNC). Full access to the JNC is available with an ASNC Membership. Join or renew today!
The March issue features a President’s Message from Dr. Panithaya Chareonthaitawee on raising awareness of amyloidosis. A featured study challenges four large language models (LLMs) with ASNC’s Board Exam practice questions, exploring AI’s potential in image interpretation and clinical reasoning. Other highlights include the I-NERVE study on [123I]-MIBG SPECT/CT in wild-type transthyretin amyloidosis, the accuracy of low-dose myocardial perfusion imaging, a tutorial on diagnosing infection vs inflammation with [18F]FDG-PET/CT, and more.
The February issue highlights a President’s Message from ASNC President Dr. Panithaya Chareonthaitawee on disparities in women’s cardiovascular care and the role of nuclear cardiology in addressing these gaps. Featured studies explore the prognostic value of transient ischemic dilation in rubidium-82 PET MPI, advancements in amyloidosis imaging with bone-avid tracers, the impact of automatic motion correction on myocardial blood flow estimation, and more.
The January issue explores the expanding scope of nuclear cardiology and the need to establish best practices for emerging techniques, as emphasized by Editor-in-Chief Dr. Marcelo F. Di Carli. Featured research includes the prognostic utility of heart rate and blood pressure response to regadenoson stress, deep learning-based motion correction for SPECT MPI, and the impact of rubidium-82 dose on myocardial blood flow measurements.
The JNC CardioConnect podcast links experts, insights, and innovations in nuclear cardiology. Join Editor-in-Chief Marcelo F. Di Carli, MD, MASNC, and authors as they discuss important articles from the Journal of Nuclear Cardiology (JNC).
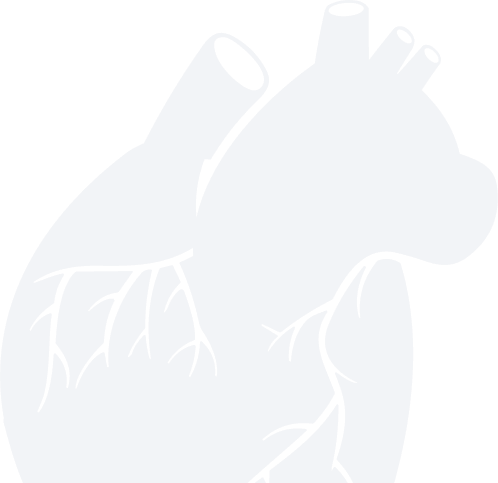
Access the latest news and updates from ASNC. Search by category of interest, date, and more.
ASNC Accepting Applications for ‘Future Leaders: The Next Generation of Nuclear Cardiology’
ASNC is now accepting applications for this year’s Future Leaders: The Next…
Step-by-Step Guide to PET/CT Interpretation in PVE
Now that 18F-FDG-PET/CT has a class 1 indication for evaluating suspected prosthetic…
F-18 PET Tracer Shows Potential for Assessing Fatty Acid Metabolism
The nuclear cardiology pipeline includes several new radiopharmaceuticals, including the novel F-18-labeled…
Sign up to receive ASNC's bi-weekly round-up of news and information related to nuclear cardiology and cardiovascular imaging.
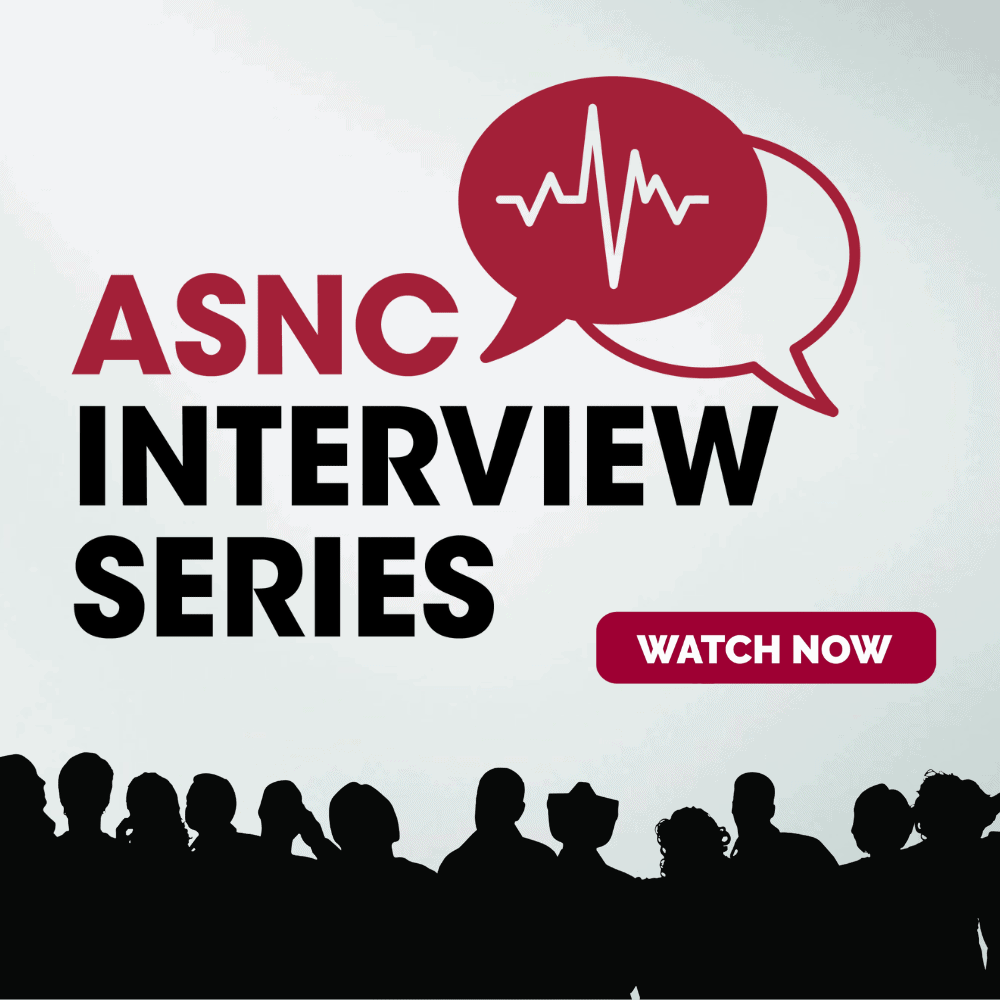
ASNC Interview Series
Explore the latest topics in nuclear cardiology through video conversations with luminaries and key leaders in the field.
Advertising
ASNC is where your marketing investment reaches the right audience and achieves optimal return.
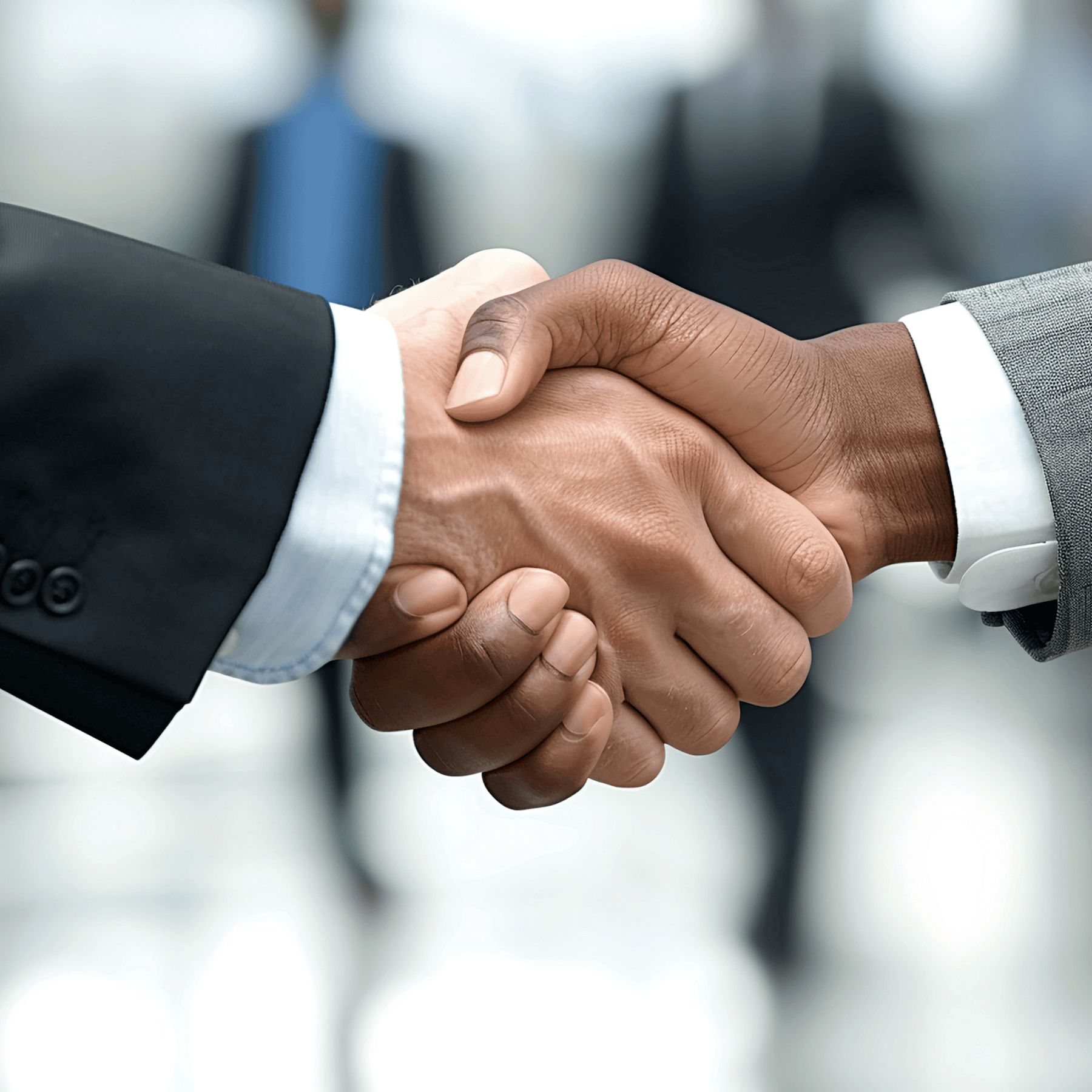